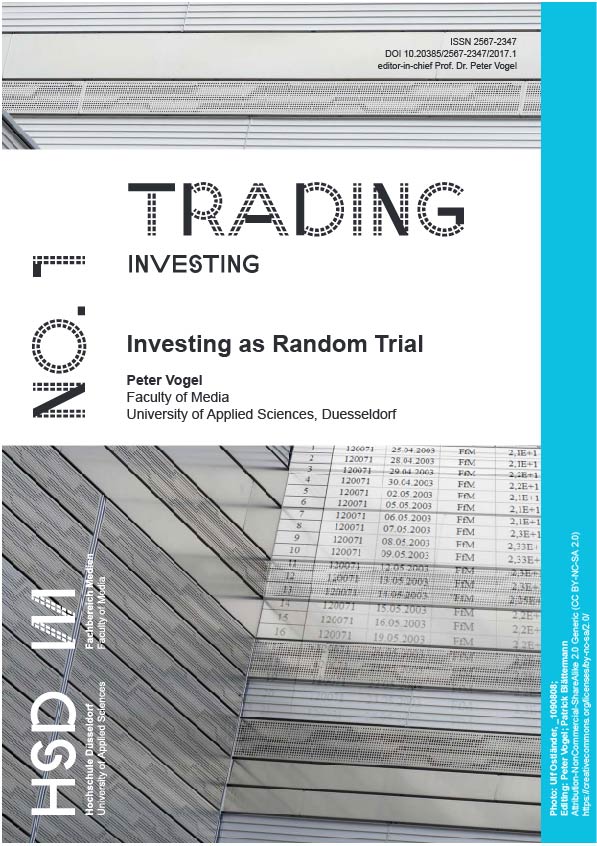
Trading
ISSN: 2567-2347
editor-in-chief: Peter Vogel, peter.vogel@hs-duesseldorf.de
staff-member: Patrick Blättermann, patrick.blaettermann@hs-duesseldorf.de
The series TRADING, founded on July, 2017, covers scientific reports about algorithmic trading.
This includes, for example, the topics investing, order execution and evaluation of stock price data.
The series intends to bridge the gap between theory and practice, reporting scientific results without secretiveness and giving new insights.
Technical terms are kept to a minimum in order to reach a broader readership.
Investing under constraints - part 1: Investing as Random Trial
An investment algorithm is introduced for a market of individual securities by maximizing the amount of investment for each trading day.
This is done under constraints in order to limit the risk and trading costs and to take into account an individual investor.
Purchased securities are selected randomly among those who meet the buy condition, making trading a RANDOM TRIAL.
The expected return is evaluated for the investment algorithm with respect to the random selection for a holding period of one day, i.e. securities are sold after one trading day.
(short abstract)
Investing under constraints - part 2: Extension of the statistics for holding periods greater than one day
The theory, developed further on in this second part, is marked as follows:
- The theory is free of assumptions about the price development of the securities. Hence, no attempt is made to establish a realistic model for price movements.
- A finite number of securities are traded, building a market.
- Investing in a security requires to meet a buy condition. After expiry of the holding period, the investment is finished.
- Trading is described by elementary recursive equations. Evaluation of the equations for the whole trading period yields the return of investment at the end of trading.The equations are excited by two exogenuous quantities:
- The number of securities for each trading day, which meet the buy condition,
- the gain, resulting from the sales of the securities after expiration of the holding period.
- Investing will be idealized in order to express the return in dependence from the exogenous quantities and investment parameters without evaluating the recursive equations. This is done by the so-called IDEAL TRADING SYSTEM.
The return of the ideal trading system extends the statistical description of part 1 towards holding periods greater than one day.
Since causality is violated by the idealizations, the ideal trading system cannot be implemented for security trading.
However, it can be simulated and is more easily quantifiable.
The effect of violating causality on the trading process and return is investigated experimentally and theoretically depending on investment parameters in detail.
(short abstract)
Investing under constraints - part 3: Extension of the statistics
In the third part of the series, the statistics in the first two parts for investing in securities is further developed.
The statistical quantities introduced thus far are gain factors/returns and the investment degree.
The new statistical quantities provide a measure of chance, risk, volatility, and trading costs.
All statistical quantities depend on investment parameters including the holding period, defining how long a new investment will be hold.
A finite set of securities is traded again and new investments in a security assumes a buy condition.
Experimental results for a market with historical price data and for a market containing random walks demonstrate the very strength of the extended statistics.
Investing under constraints - part 4: Extension of part 3
The fourth part of the series "Trading" presents experimental findings of price data statistics for a holding period of one day.
The statistics include gain factor, return and investment ratio of part 1 as well as volatility, chance, risk and trading costs of part 3.
Buy conditions are defined by means of the price relationship for two subsequent trading days, as in part 1.
The experimental findings for the market FEBRDUSA_1 of historical price data provides a coherent description of the influence of the buy condition on future prices.
The maximum return is provided by a buy condition describing a price slump.
Since the statistics incorporates the limited amount of new investments, exaggeration due to arithmetic averaging is avoided.
The experimental findings for a market of random walks confirm that there is no dependence on the buy condition in this case.